Substance over style
In this article, Machine Learning Program’s (MLP) Head of Product, James Parry, looks at how the insurance industry can make use of machine learning tools and, more importantly, how to pick the right tools for the job.
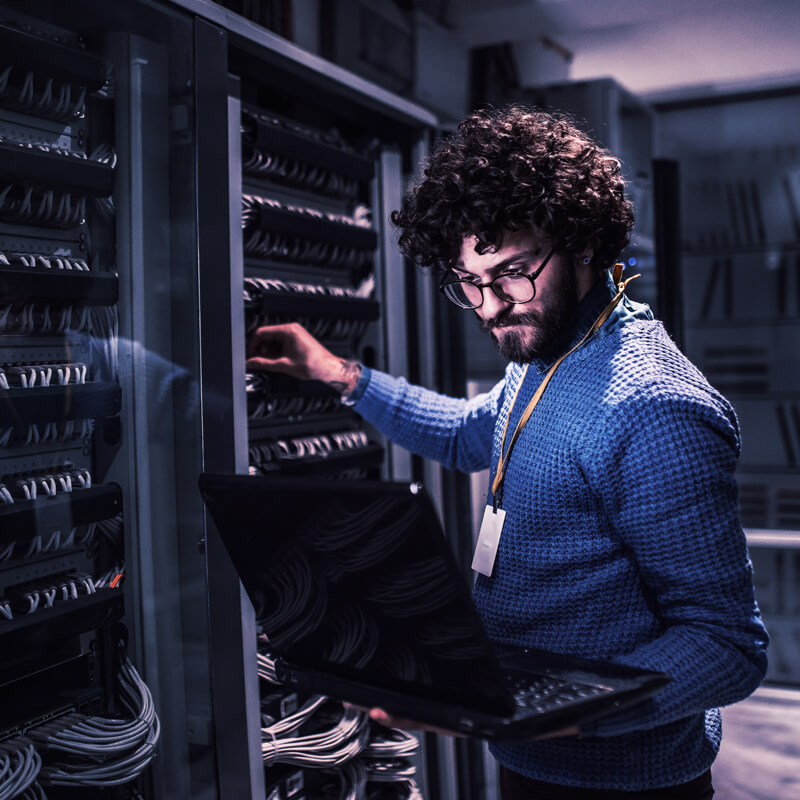
Machine learning has been around for a while and is widely used from YouTube hobbyists to the Silicon Valley tech giants. However, while the adoption of applied AI in the insurance community is still very much in its infancy, wherever you look you can’t avoid the wonderful new applications of artificial intelligence.
AI is being used to generate new chapters of Harry Potter books and create three-dimensional talking heads based on little more than a couple of still photos. I have seen what I will look like when I’m 90, and what the computer thought I looked like as a toddler (it was pretty close if I’m honest!). Gone are the days of computers beating humans at chess, four years ago DeepMind’s AlphaGo was beating world number 1 ‘Go’ player Ke Jie. Last year ‘Quake 3 Arena’ became the battlefield (quite literally) as AI ‘capture the flag’ players outperformed their human counterparts. But as fascinating as these exploits are, is there any substance to it?
Well, yes. The same techniques developed to predict the behaviour of an opponent in order to improve the performance of a player can and are applied to improve the performance of just about anything. Whether we’re looking at drug trials, autonomous vehicles, or general insurance, one of the fundamentals of machine learning is the ability to interpret risk. In the same way that reinforcement learning rewards children for positive behaviour and penalises for poor performance, so too can machines ‘learn’. Given a new medicine, a stop sign or an insurance request, based on knowledge learnt to date, what is the risk of being rewarded or penalised for the decision?
Why not therefore apply artificial intelligence directly to improving business models within financial markets, medical research, or insurance?
Evaluating risk could get even easier
The reality is, applied AI is becoming commonplace in many industries and the sub-industries of FinTech, MedTech and InsurTech are awash with companies offering machine learning products.
Looking at general insurance, when a person requests a quote for a vehicle, the accepted industry term for the individual/vehicle combination is a risk (and we know that machine learning is about evaluating risk). It is the insurer’s job to price this risk based on several factors. Most of us have filled in a form at some point asking for the age of the driver, the make and model of the vehicle etc. Experience has also taught us that younger drivers are more likely to claim, and to mitigate the claim it would be wise to increase the premium or excess to mitigate costs. It is generally accepted that ‘age’ is just one of 15-20 key rating factors for determining how to price a risk.
But what if age was no longer the key predictor we once thought it to be? What if we had the ability for a machine trained thousands of times, on millions of records, with hundreds of columns to advise on:
• Whether a given risk is likely to claim or renew?
• The likelihood of an applicant or a claim being fraudulent?
• What is the ideal price to quote based on the last 5 minutes of market activity?
Machine learning technology has been widely adopted with varying success on website chat bots and mobile applications that allow users to submit pictures of their valuable items or assess the damage of a road traffic accident (RTA). However, the industry has only recently begun to apply machine learning to the core business – the policy itself.
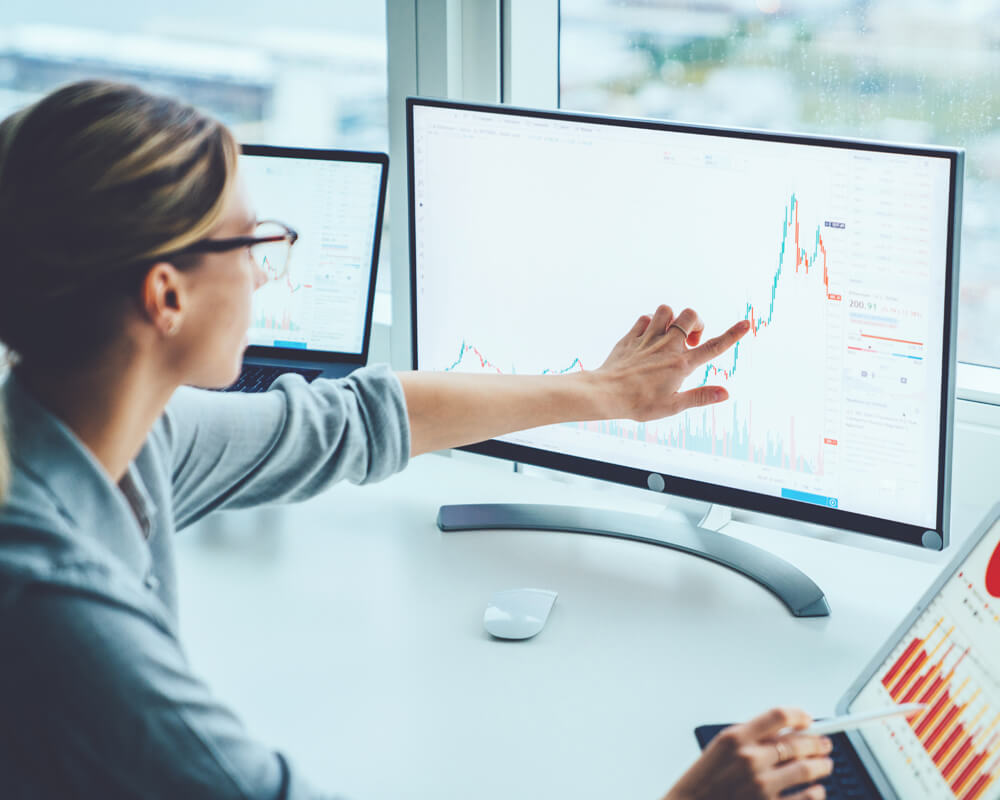
Moving with the times
The databases, pricing applications, enrichments and connectivity that underpin today’s modern insurers are not just tools of the industry, they are a fundamental necessity. To gain a competitive edge, businesses need to ensure they have the best tools at their disposal and the successful companies that have already embraced machine learning into their tech-stack are beginning to see real wins.
With machine learning for insurtech still in its infancy, it goes without saying that once the business driver has been identified, a carefully considered decision will need to be made on what tech to deploy. It can certainly seem like a jungle with all the neural networks, decision trees and random forests to navigate. So how to decide?
One should be comfortable with the quality and integrity of their chosen data science team. The true substance of an AI lies in the quality of its creator; the data scientist who knows not only the latest software and how to write efficient code, but who also understands the subject matter and the meaning of the data. The second key component in the decision is the data itself:
• What data can be used to train on as well as run with?
• Will the end result have been trained on public data, your own data, or a combination?
• What external public and private enrichment will be available?
• How will the model be kept up to date to avoid drift?
• Does it all adhere to the most stringent regulations?
With all this considered, will the end result deliver actionable insight to improve the bottom line of your business? If it does, whether the end result looks visually absorbing or not, you are on the right path.
Machine learning tools are fantastic at performing given tasks. Trained and delivered well, a single AI tool can be used to enhance risk selection and turn a profit. Combining multiple AI tools to predict price, assess risk and detect fraud, while continually evaluating performance and retraining…well, that’s just the fundamental necessity of tomorrow’s insurer.